Metrics: How to Improve Key Business Results Part 16
You’re reading novel Metrics: How to Improve Key Business Results Part 16 online at LightNovelFree.com. Please use the follow button to get notification about the latest chapter next time when you visit LightNovelFree.com. Use F11 button to read novel in full-screen(PC only). Drop by anytime you want to read free – fast – latest novel. It’s great if you could leave a comment, share your opinion about the new chapters, new novel with others on the internet. We’ll do our best to bring you the finest, latest novel everyday. Enjoy!
Figure 8-6 shows an example of what I estimate to be the performance norm for the unit.
Figure 8-6. Letting the data determine the norm At this point, I would ask the department if the chart (Figure 8-6) seems correct.
"Would you agree that your customers' expectations lie between a 75 percent and an 85 percent rate of performance?" I usually get an affirmative nodding of heads. That isn't enough, though. I want to get as close to correct as possible, but I'm willing to accept what amounts to a good guess to start. I say this because regardless of where we set the expectations, we must stay flexible regarding the definition. We may obtain better feedback from the customer. We may change our processes in a way that the expectations would have to change (rarely do customers grow to expect less-most times they will only expect more).
I ask, "So, would the customer be surprised if you delivered over 85 percent? " If I get a yes, I ask the logical follow up question, "How did the customer react in February of Year 2 and August of Year 3?" And, "How did you achieve these results?" I'd ask the same questions about March of Year 3 and August of Year 2. Depending on the answers I might readjust the expectations.
I mentioned that you could use statistical a.n.a.lysis. Let's look at an example. Figure 8-7 shows the same data a.n.a.lyzed using Microsoft Excel's Data a.n.a.lysis Add-in tools.
Figure 8-7. Histogram of the two-year sample Based on the histogram, my guess of 75 to 85 percent would seem acceptable. More exact may be greater than 75 percent and less than or equal to 85 percent. If we look at a simpler view of the data spread, we find that the range offered is quite reasonable and probable. But again, the main test will be to check our ideas against customer feedback.
A simple frequency chart of the same data, shown in Figure 8-8, tests the visual interpretation and affirms the guess.
Figure 8-8. Frequency chart of two-year sample.
Recap.
The existing schools of thought on performance measures-specifically the concepts of targets, thresholds, and using measurements as goals-should be replaced by a collaborative approach that gives owners.h.i.+p of service and product quality to the workforce. This is accomplished by determining customer expectations.
Expectations are almost always a range.
Meeting expectations is what you want to do on a regular basis.
Failing to meet expectations is an anomaly that should be investigated to determine causes.
Exceeding expectations is an anomaly that should be investigated to determine causes.
Positive and negative anomalies should both be of concern to the organization. Whether you are exceeding expectations or failing to meet expectations, you need to investigate further.
When setting expectations, look to the data to help identify the norm. The question then becomes, "Is the norm equal to the customers' expectations?" If yes, then the data should reflect that. The purpose is to be able to identify the anomalies that fall out of the norm or outside of the range of expected behavior.
Treasure anomalies-because anomalies are where your metrics earn their return on investment. The main reason to adjust the range of expectations is to ensure that you are properly identifying the anomalies.
Conclusion.
The old school-of-thought doesn't work. Using metrics as a form of motivation falls closer to manipulation than collaboration. You'll need to develop a solid rapport with your workforce and fully team with them to use metrics properly. Setting stretch goals, targets, thresholds, using measures as goals, and rewarding reaching a measure as an incentive are examples of using the wrong tool for the job.
Meeting customer expectations is the real goal. Anomalies to the expectations can provide useful information.
Expectations provide a clear context and are the key to open the doors to improvement.
Creating and Interpreting the Metrics Report Card.
I struggled for a long time trying to decide how to introduce the Report Card to you. I debated if I should present it as another tool (like the Answer Key), or offer it as a methodology. What I settled on was to offer it as a real-life example of how metrics, when used within the constructs I've offered, can evolve and take shape.
I will relate to you the three-year journey to develop a viable metric program for an organization in which I had to clearly articulate the overall health of the organization and the health of each of its core services. I made mistakes along the way, learning, changing, testing, and trying. I will share with you the journey of discovery and the final destination I arrived at. Hopefully you can learn from my mistakes and benefit from the final product as either a template or example for your use.
The top-level executive asked a straightforward question of our CIO, "How healthy is your organization? And how do you know?" This fit in well with the curiosity of my CIO, who wanted meaningful information about the organization's health, but didn't know how to communicate the need.
With this root question in hand, I endeavored to find a way to build a metric program that would provide meaningful answers. The "we" I refer to is a metric project team made up of Ernst & Young consultants and myself.
To instill a metric program in an organization that was not truly ready for it was a difficult proposition. One that was actually impossible to succeed at. I say that with all humility because even with the level of success we enjoy today with the Report Card, it's still not used to its full potential. But we are getting better, and the Report Card has lasted longer than any other reporting tool our organization has used to date.
To succeed at this challenge while keeping to the principles and values I've laid out so far in this book was even more difficult. To make this possible, especially when working with a mandate (perhaps one of the worst ways to implement any specific improvement effort because it removes the chance for "buy-in" of the workforce), required a metric program that would have benefit to the data owners, the executive requesting the answers, as well as all the people in between.
Along with a metric system that would provide meaningful information to all levels, I wanted it to be easy to understand and require as little translation between levels as possible.
As I've written earlier, you can't do this on your own. It would have been impossible for me to succeed at this by myself-not only because the effort was too large for one person, but because I wouldn't be able to obtain buy-in on my own. The service providers wouldn't buy in and believe how the information would (and wouldn't) be used if I didn't involve them throughout the process. Management wouldn't buy in to the idea that whatever solution I developed was going to work, since I was already a member of the village. No prophet (or metrics designer) is accepted in his own village. So I ended up with a team of outside consultants, myself, and heavy involvement of each service provider.
Concept.
First, you may be thinking, "Why a Report Card and not a Scorecard? After all, many organizations track metrics using them." I started with the thought of using a scorecard. The scorecard methodology included using measures from different areas (which fit the concept of triangulation), but the areas were not even within the same family of measures (see Answer Key). The other problem was that Scorecards mixed in the more "risky" efficiency measures along with effectiveness ones. Without a doubt, I wanted to stay in the Service/Product Health area, since I'd be working hard to break through enough barriers without fighting the war that would ensue with efficiency measures.
What I liked about the scorecard (and dashboards) was the combination of measures to tell a fuller picture. That fits the definition of a metric rather than just a bunch of measures.
But think about the name itself: the scorecard represents a means of knowing "who's winning." In the fall of 2011, I watched the University of Notre Dame lose its opening football game 2320 to the University of South Florida. What was amazing was that Notre Dame "won" in every imaginable category, and not by just a little. Offensively, defensively, all categories except one: turnovers. In the end, the only statistic that matters is the final score, and that is determined by points. Those points are normally predictable through other measures.
So, the scorecard will tell you quickly who is winning, and who has won. It won't tell you, though, who is performing better in specific areas. A fan may only be concerned with the scorecard, but I was working with the equivalents of the coaching staff. The offensive, defensive, and special team coaches, along with the running backs, receivers, and linebackers coaches would all want to know how their units were performing.
I wanted the best of both. I wanted a final score and indicators of the quality of performance that led to that score.
What we came upon is the concept of a Report Card. This metric would be like a college report card, where the student receives feedback throughout the year on how well his education is progressing. There are quizzes, tests, and papers to be graded. At periodic intervals (mid-semester and at the end of the semester) grades are levied. Based on the feedback the student knows if he is doing well, if he needs to improve, or if he is exceeding expectations.
In each case, the organization, like the student, has decisions to make, based on the entire report card. It's not enough to celebrate the exceptional grades (A+) and denigrate any poor grades. It requires more information before a decision can be made. Was the A+ obtained at the expense of a different subject? What is the benefit for the A+ over an A or even a B? Is the poor grade in a subject that the student needs/actually wants? Can the cla.s.s be dropped? Is it required for the major?
For our purposes, we treated the metric we developed in the same way as a report card. While we may be happy to get higher-than-expected grades, we only want the following results: Those that don't require more than an acceptable effort (for example, if the student is neglecting other subjects or the student is burning the midnight oil to the point that his health is suffering).
The student obtained the grades without "cheating." In the organizational context, cheating equates to doing things outside the acceptable standards, policies, or processes.
The student earned the grades. We don't want grades that were not earned-positive or negative. The metric should reflect the performance of the service from the customers' viewpoints.
When we look at grades that fall below expectations (the failure of a quiz or test, for example), we also have to ask the following: Was the failure due to an avoidable circ.u.mstance? This might include poor study habits, not doing the required work, or a lack of prerequisite coursework?
Was it due to a lack of effort or focus?
Is the subject actually required for the major? If not, is it a course that can be dropped? If the service is not part of the organization's core services, can it be dropped? Are results "below expectations" acceptable?
Let's look at how the Report Card is built, from an individual service to the overall organization's health.
Ground Work.
All organizations have customers. In our case, to answer the questions posed by our executive leaders.h.i.+p, we chose to answer it in the context of how well we delivered our services to our customers. We could have taken the question to mean how efficiently we were producing our products and delivering our services...but using the Answer Key we chose to start at the more critical focal point of how well we were satisfying our customers (Service/Product Health). This focus allowed us to design a program from the bottom up.
By focusing on the customer's viewpoint (effectiveness), we found it possible to introduce the metrics at the staff level, mitigating the fear, uncertainty, and doubt normally encountered with metrics. We were able to a.s.sure the workforce, including supervisors, middle-managers, and directors, that the information we were gathering, a.n.a.lyzing, and eventually reporting would not reflect how well any one person in the organization was performing, nor any unit. It would instead communicate how the customer saw our services and products.
The Information would be valuable to everyone at every level. Without "blame" being involved, we could address the customers' concerns without distraction. Not only wasn't blame ("who" wasn't important, only the "why"), but the information we had wasn't considered fact-it was the customers' perception that mattered more than any argument about fact.
This helped address the normal arguments against collecting certain data: "But we have no control over that," and "It's not our fault."
We agreed to use the following major categories of information suggested by the Answer Key: Delivery.
Usage.
Customer Satisfaction.
We further agreed that Delivery, for most services, would be further broken down into Availability, Speed, and Accuracy. This was especially useful since the most contentious measurements resided in the Delivery category. Most of our service providers were already collecting and reviewing measures of Customer Satisfaction through the trouble call tracking system surveys. Usage was a "safe" measure since rarely does anyone consider usage numbers a reflection of their performance.
Since we expected the most pushback from the Delivery measures, we hoped to ease the resistance by using the three areas just mentioned (triangulation).
So, the root question, and our higher-level information needs were identified easily. Of course we still had to then identify individual measures and their component data. Before we did this, we needed to know which services would be included in the report card. We needed to know the organization's key/core services. A service catalog would have helped immensely, but at the time, this was lacking. In many organizations suffering from organizational immaturity, much of the prerequisites for a solid metric program may be missing. This is one of the reasons that using a metric program is considered a mature behavior.
While trying to implement a mature behavior (like a metric program) in an immature organization can be rife with problems, if done carefully it can actually act as a catalyst for moving toward maturity. In our case, the metric program clearly helped the organization think about and move toward developing a service catalog. While it had been identified as a need long before the metric program was launched, the metric effort helped focus on the deficiency.
Another behavior encouraged by the Report Card would end up being the more consistent and accurate use of the trouble call tracking system. Since a large amount of data (for almost all of the services included in the Report Card) relied on this system, the organization had to become more rigorous in its use of the system.
Until the organization doc.u.mented a service catalog, we did our best to identify the services that would be considered core by the majority of the organization.
Being an information technology organization we had a lot of services and products to choose from. Some of the key services we chose included the following: Service Desk.
E-mail.
Calendaring.
Network.
Telephone.
These services were easily accepted as part of the core services we provided. For each of these services, we built a template for collecting measures in the areas identified. This template would include the service, the type of information (category) to be used in the a.n.a.lysis of the health of the service, and specific measures that would be used to answer the root question. The results are shown in Table 9-1.
You may have different services to look at. You may be, for example, in an entirely different industry than the one I'm using in the example. Even if you have the same services, you may have different categories of information and therefore different measures.
I'm going to focus on only one area, Service Desk, to continue this example, but I want to first explain the "not applicable" measure for usage of e-mail. Our organization provided the e-mail for our customers-basically as a monopoly. Our customers had no other choice for their work e-mail. They could use other e-mail services (Gmail, for example), but it would have to go through our system first and then could be auto-forwarded. So we started with "not applicable" and didn't measure usage for e-mail. We also did not measure usage for Calendaring, Networking, and Telephone, for the same reasons. While we didn't use a measure for usage in these cases, there are measures that could have been used.
For e-mail we could have used Percentage of Use and the Number of Customers who Auto-Forwarded their e-mail to a different e-mail provider.
For Calendaring we could look at Frequency of Use, and Percentage of Features Used. Calendaring was a new enough service that it would have been worthwhile to know the level of usage (acceptance by our customers) of the features.
Measuring the Frequency of Use of the internet and telephones still seems meaningless since they are high-use services that we held as a monopoly.
The following are two points that I want to make clear: Some of the measures can be "not applicable." Although triangulation dictates that you attempt to have at least three measures from different viewpoints, it is not mandatory.
Just because you can think of measures, doesn't mean that you have to use them.
Just because you can think of measures, doesn't mean that you have to use them.
So, back to the Service Desk. Let's look at the specific measures which were identified for this service.
As explained in Chapter 5, the Delivery measures of Availability, Speed, and Accuracy would be measured objectively through information gathered from the service provider. The great news for us was that the manager of the department was (and still is) an extremely compa.s.sionate leader. Where much of the dangers and warnings I've written about come from fear, uncertainty, and doubt-the realization of those trepidations occurs most times because the manager misuses the metrics. In the case of our Service Desk, I didn't worry that the manager would misuse or abuse the information we provided. If I told her the data showed that a worker was negligent, lazy, or incompetent, rather than take premature action, she'd investigate to first find out the "truth" behind the data. Then if the interpretation I offered was accurate, she'd work with the staff member to address the issues-compa.s.sionately.
The manager's att.i.tude made the Service Desk an excellent service to start with. We had a high confidence level in her ability to help us sell the program to her staff and become a solid example for other service providers.
Another positive from starting with this service provider was the large amount of data easily attained. The Service Desk was the highest user of the trouble call tracking system. The majority of the data would be through this system. The Availability data (abandoned calls) was available through a totally automated call system, making it an objective set of measures. Customer satisfaction was handled by a third-party survey organization. So, this service provider had all the data we could want and most of it was through objective collection methods. All of it was obtainable without intruding on the departments' day-to-day operations. An ideal service to start with.
Speed would be tracked with a little less objectivity than Availability. Since it was based on the speed of resolution, it would be derived from the time to open and time to close trouble-call cases. This required that the a.n.a.lyst answering the call logged in the call accurately at each phase-opening and closing. Since the manager believed in metrics, she encouraged (and ensured) the workers logged cases accurately.
Accuracy was also measured using the trouble-call tracking system. Rework was defined as cases that were prematurely (incorrectly) closed. We could try to identify errors (defects) throughout the solution process, but since much of finding solutions to customer problems included trial and error, this was not an easy place to start. Even with the manager's compa.s.sion, I didn't want to ask the a.n.a.lyst to track how many different guesses they tried before they got it right. This would require a high level of trust (for the worker to not believe it would reflect about herself), a trust I hadn't developed yet. Another problem with this possibility was that the information might not reflect the customer's view point. Most customers expect a fair amount of trial and error, and hunting around for the right solution.
In each case-Abandoned Call Rate, Time to Resolve, and Rework, the data would reflect the customer's view point.
Moving from Delivery to Usage was, in this case a more difficult set of measures. Since the Service Desk was an internal service provider (the customers were not paying for the service), usage did not reflect income to the organization. The manager had a very healthy big picture view of the mission of her department. If usage was "high," that might reflect the instability of the IT systems, services, and products. It may also reflect the ineffectiveness of IT-related training. In most businesses and services, high usage would be a good thing. In the case of the Service Desk, high usage might be as bad as low usage. Also, if the usage was "low" it wasn't clear if this reflected that the training was excellent, and the systems stable or that the customer didn't find the Service Desk a meaningful, useful provider.
As with most measures, the information derived from it would have to be interpreted. Further investigation should be carried out rather than jumping to any conclusions. Any extremes could be a negative in this case-but the information was still useful. Since we had data, measures, and information from a decent span of time (over three years), we could determine if there were changes in the customers' patterns of usage. By looking at unique customers, we could determine if there were anomalies-up or down. These spikes may not indicate a good or bad thing. Instead they would indicate only that something had changed.
To be meaningful, I had to have longitudinal data for comparison; otherwise anomalies could not be identified. In some cases it isn't as important-but in the case of usage, it was. The key is to be able to determine anomalies-measures that fell out of the range of normal expectations. After we identified the proper measures and data points, we had to identify expectations.
The task is to identify anomalies-measures that fall out of the range of normal expectations.
This highlights an important facet of the metric program. Your information is only indicators and shouldn't be acted upon without further investigation. Rather than expect these metrics to be the impetus for action, it more often is the indicator that something is amiss, something has changed, or something needs to be investigated.
Metrics: How to Improve Key Business Results Part 16
You're reading novel Metrics: How to Improve Key Business Results Part 16 online at LightNovelFree.com. You can use the follow function to bookmark your favorite novel ( Only for registered users ). If you find any errors ( broken links, can't load photos, etc.. ), Please let us know so we can fix it as soon as possible. And when you start a conversation or debate about a certain topic with other people, please do not offend them just because you don't like their opinions.
Metrics: How to Improve Key Business Results Part 16 summary
You're reading Metrics: How to Improve Key Business Results Part 16. This novel has been translated by Updating. Author: Martin Klubeck already has 1253 views.
It's great if you read and follow any novel on our website. We promise you that we'll bring you the latest, hottest novel everyday and FREE.
LightNovelFree.com is a most smartest website for reading novel online, it can automatic resize images to fit your pc screen, even on your mobile. Experience now by using your smartphone and access to LightNovelFree.com
- Related chapter:
- Metrics: How to Improve Key Business Results Part 15
- Metrics: How to Improve Key Business Results Part 17
RECENTLY UPDATED NOVEL
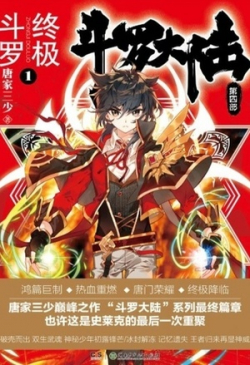
Soul Land IV (Douluo Dalu) : Ultimate Fighting
Soul Land IV (Douluo Dalu) : Ultimate Fighting Chapter 1000: Space Origin Crystals At Last View : 817,085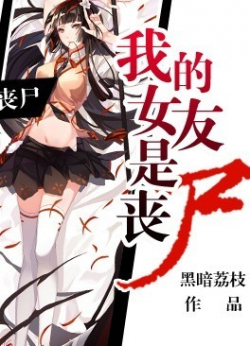
My Girlfriend is a Zombie
My Girlfriend is a Zombie Chapter 868: Ive Given Up on Treatment View : 2,318,809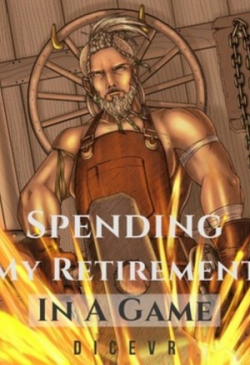
Spending My Retirement In A Game
Spending My Retirement In A Game Chapter 921 The Dark Forest View : 1,196,054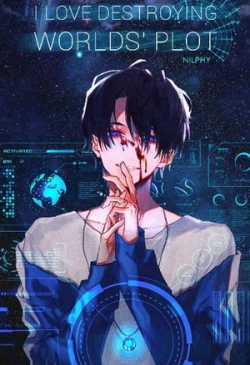
I Love Destroying Worlds' Plot
I Love Destroying Worlds' Plot Chapter 1542 20.106 First Black Star - Yes Qinjing's Rebirth [End] View : 519,083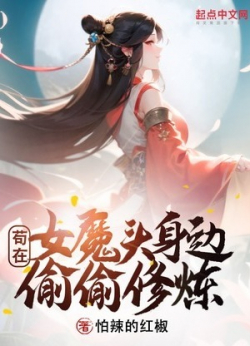
Cultivating In Secret Beside A Demoness
Cultivating In Secret Beside A Demoness Chapter 1365: The Demoness and Dongfang Xian'er Meet (1) View : 555,751
Raising My Children With My Personal Spatial Ability
Raising My Children With My Personal Spatial Ability Chapter 1860: Are You Hungry? View : 1,289,677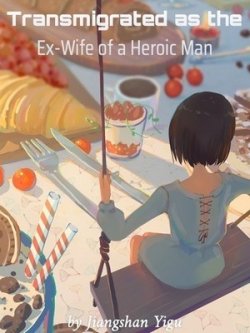